The Voices of Money20/20: A Scienaptic AI Two-fer
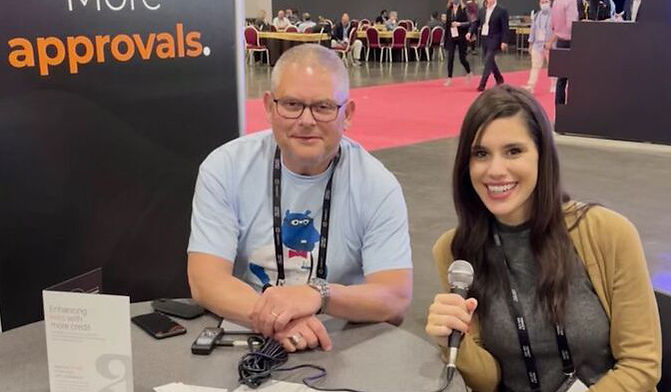
Dec 7, 2021
Finopotamus was onsite at Money20/20 USA in Las Vegas. Co-founder John San Filippo interviewed more than two dozen technology experts on a wide range of topics. The results of these interviews are presented in this series called “The Voices of Money20/20.”
For this installment, Scienaptic AI’s President Pankaj Jain and Client Success Executive Dakota Patsfield sat down with Finopotamus to discuss artificial intelligence (AI) driven loan decisioning.
“Our company provides an AI-powered credit decisioning platform,” Jain told Finopotamus. “It helps lenders find those ‘diamonds in the rough,’ allowing lenders to say yes to more borrowers, instantly and with complete confidence.”
Finopotamus asked Jain and Patsfield to describe current underwriting methodology at the typical credit union as a way to level-set the discussion.
“If you’re a credit union, you have a loan origination system (LOS) and a core processing platform,” said Jain. “Regardless of what channel it comes through, the application is captured by the LOS, which then pulls the applicant’s credit bureau report. The credit bureau sends over some 1,500 attributes, but the LOS strips that data down and considers eight to 10 attributes.” Jain said these include basics like income, charge-offs, bankruptcies and number of trade lines.
Jain added that whether the application is passed to a human underwriter or an automated decisioning system, the result is the same — loan decisions are made based on a tiny, albeit important, subset of the available data for any given applicant.
The AI Difference
“With our decisioning platform, 100% of loan decisions can be automated,” said Jain. “That’s because we’re able to quickly analyze all of the data from the credit bureau and marry it to alternate data from sources like LexisNexis or MicroBilt. Ultimately we end up looking at about 5,000 different variables.”
“We're actually able to tap into that same credit bureau data the institution is already paying for and segment the borrowers into structured buckets and find organized patterns of data,” added Patsfield. “We bring more value to that credit bureau expense.”
By considering these variables, the system might determine, for example, that one particular individual with a 680 FICO score is more likely to behave like someone with a 740 FICO score, according to Jain.
Just as important is the fact that this detailed analysis does not slow the process down. “It literally happens in the blink of an eye,” said Jain. “Our system returns a decision in 200-300 milliseconds.”
Jain told Finopotamus that Scienaptic AI offers its system as either a cloud-based or on-premise solution based on the wants and needs of the institution, but most customers choose the convenience and reliability of the cloud. “We operate in a secure cloud on AWS (Amazon Web Services) in the United States,” said Jain. “We’re a SOC 2-compliant organization. Our infrastructure is probably more secure than that of many credit unions.”
Better Decisions
Jain said that with margins so tight, credit unions are forced to be conservative in decisioning as a means of avoiding excessive loss. “Most of the credit unions we are working with operate at less than 25 basis points,” he said. “That's why the look-to-book ratio at many credit unions is about 30-35%. It’s very low compared to the industry.”
Jain added that while Scienaptic AI’s system can help boost the number of loan approvals without increasing risk, it can also catch riskier loans that may have otherwise been approved.
“We had a recent example where we declined an auto loan application. The applicant had a FICO score of 720 or 730 and the debt-to-income ratio looked fine,” said Jain. “The credit union overruled our decision and made the loan. Guess what? The automobile ended up confiscated by the police and, of course, the payments stopped coming in. By pulling in LexisNexis data, our system knew that the borrower was a repeat felon.”
Patsfield also noted that better loan decisions lead to better member retention. “If credit unions are approving more loans, they’re not losing business to someone else,” she said. “That also means that member is more likely to come back to the credit union the next time they need a loan.
“By merging all this data, we really get a 360-degree view of the applicant,” she continued. “It allows credit union lending to become deeper, sharper and stronger.”
Measuring Success
Finopotamus then asked how a credit union that deploys the Scienaptic AI platform measures success.
“After the contract is signed, there is an onboarding process, which takes six to eight weeks,” said Jain. “We bring in a lot of quick-start models for each product, but we also ask the institution to provide us with two or three years of loan application data, as well as performance data on approved loans.” Scienaptic AI then runs that data through its system, which allows the institution to compare actual results to projected Scienaptic AI results. In other words, the credit union has a good idea what to expect before it ever goes live on the system.
“Assuming there haven’t been major changes in the product mix or member profile, they’re going to know what to expect in terms of business performance before they ever go live,” said Jain. “At one recent credit union, we saw approvals jump from 61% to 81% overnight.”
“We love the way our technology gives credit unions the ability to support their communities,” added Patsfield. She said making a loan to a member who might not have otherwise been approved is a success that can’t be measured in just dollars and cents.
Looking Forward
Credit unions have money to lend, according to Jain. “We did some research and learned there’s about $2 trillion of deposits at U.S. credit unions,” he said. “They serve about 125 million members across the country and loan-to-deposit ratios average 68%. That means there’s about $800 billion sitting in credit unions that could be loaned out.”
According to Jain, loan decisioning is changing for the better and credit unions that do not adopt that change put themselves at risk. “If credit unions hope to compete with big banks and fintechs, they need to get better at what they do. They can either push forward with new technology or be left behind,” he concluded.